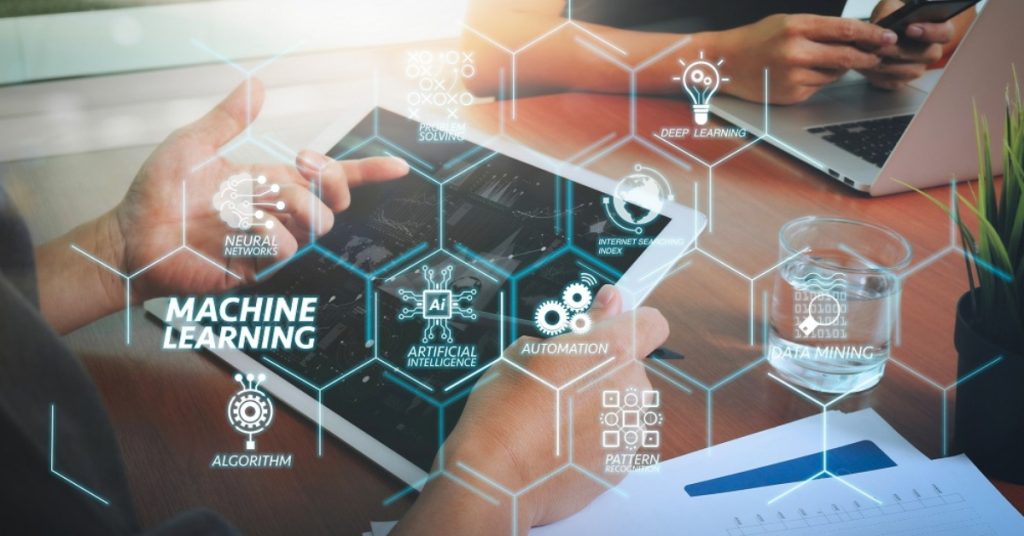
In a job interview, IT professionals and business experts can effectively showcase their machine learning (ML) knowledge by clearly articulating how ML contributes to business goals.
Understanding the standard process for building machine learning models is essential for an ML engineer. This includes using test and training datasets, introducing new datasets, fitting models, and striving for convergence. For instance, professionals should be familiar with the labor-intensive process of creating test and training sets, which may involve manual web scraping—a task few enjoy.
Awareness of major pitfalls in machine learning is crucial. One common issue is fitting or dimensionality problems, leading to complex and unhelpful programs for enterprises.
Proficiency with neural networks is also vital. Professionals should understand both classical algorithms and how weighted inputs are used in modern AI/ML systems to produce accurate results. They should also know how to manage bias and variance and effectively move toward model convergence.
Additionally, professionals must be knowledgeable about ethical and explainable AI/ML. In today’s era of rapid innovation, it’s important to address the “black box principle,” where the operations of an ML program are opaque to humans. The explainable AI movement aims to resolve these issues and ensure ethical AI/ML applications in various industries.
Comments (0)